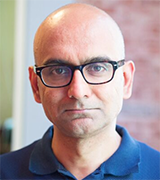
Truth in measurement has never been more important than it is today. Therefore, truth is our only agenda. But arriving at that truth has never been more complicated. While many view big data as a panacea for measurement in a digitally rich world, we know it’s not that simple.
Nielsen’s panels have been the foundation of persons-level measurement for decades, and they remain so today. The growth of big data, however, can’t be ignored as a source of valuable information. But big data alone isn’t suitable for representative measurement. Think about when you change the channel on your TV. That change becomes part of big data, but there is no record of who made the change or who witnessed it.
To highlight the shortcomings of big data from a measurement perspective, we conducted an analysis in the U.S. earlier this year that compared set-top box data with set-top box data that we calibrated with Nielsen panel data. The analysis found that the uncalibrated data is inherently biased and underrepresents minority audiences.
That’s not to say, however, that big data has no value. Quite the opposite. But it does need to be grounded in a foundational truth set. That’s where our panels and artificial intelligence (AI) come into play. Our panel data—the key to persons-level measurement—is the perfect truth set for training big data.
Through the application of AI, we use big data to dramatically broaden our measurement capabilities while preserving quality and representativeness. Today, AI is integral in our measurement methodologies. For example, it played a pivotal role in the development of our enhanced measurement capabilities for local TV markets, which combines the scale of big data (return path data {RPD} from TV sets) with fully representative in-market panel data.
As we sought to integrate RPD into our local measurement, we identified four key uses of AI.
Recognizing Data Patterns
As we researched ways to integrate RPD into our measurement, we identified limitations associated with the RPD through what we refer to as “common homes analyses.” For these analyses, which continue today, we compare tuning data from Nielsen meters with RPD tuning data. These analyses cover more than 5,000 homes (12,000 TVs) each month and have found that RPD misses some tuning.
To address this shortcoming, we developed a patent-pending technique that uses classifiers to recognize the patterns associated with the missing tuning in RPD homes. From there, AI algorithms remove these homes from use in measurement.
Knowing When Set-Top Boxes are On and TV Sets Are Off
Nielsen’s common home analyses analyze more than 77 million minutes of tuning in a given month, which provides powerful insights. That tuning, however, is not always accurate. For example, people don’t always turn off their set-top-boxes when they turn off their TV sets. The RPD presents these situations as TV viewing even though no one is watching.
We can overcome this limitation by employing deep learning classifiers to identify situations where the set-top-box is on while the TV is off. The algorithm then removes the invalid tuning from the RPD.
Identifying Household Characteristics and Demographic Information from RPD
RPD is nameless and faceless, and it can’t provide demographic information. Demographic information is critical in correctly representing all segments of a population. And beyond that, accurate measurement means being able to measure people, not just households.
So to unlock the powerful information within RPD homes, we calibrate RPD with the known characteristics, demographics and tuning information from more than 45,000 Nielsen metered homes and third-party assigned characteristics and demographics. We then add these inputs into a patent-pending recurrent neural network and mixed integer programming technique that accurately identifies the characteristics and demographics of RPD homes. This AI algorithm allows us to accurately report demographic characteristics of the persons and households.
Determining Set-Top-Box Room Location
Nielsen panel information provides viewer information and viewing location. RPD does not provide either. We can, however, obtain that information from RPD through AI. We use a scientifically-proven methodology to identify which household member is watching and where viewing is occurring within the home.
Research has found that room location is one of the key predictors of which household members are in a viewing audience. So we use a classifier to identify room location of the set-top-box where tuning is happening in RPD homes. That way, we can use this variable in the viewer assignment process.
With so much information available today, it’s tempting to view big data through rose colored glasses. Without a connection to persons, however, big data is far from accurate. We found AI as a powerful way to address bias in the big data and delighted to bring the innovation to the clients. This innovation benefits from both the wealth of information that big data sources provide and a truth set that ensures that they can plan, activate and measure based on data that is representative and accurate.
This article first appeared on Medium.